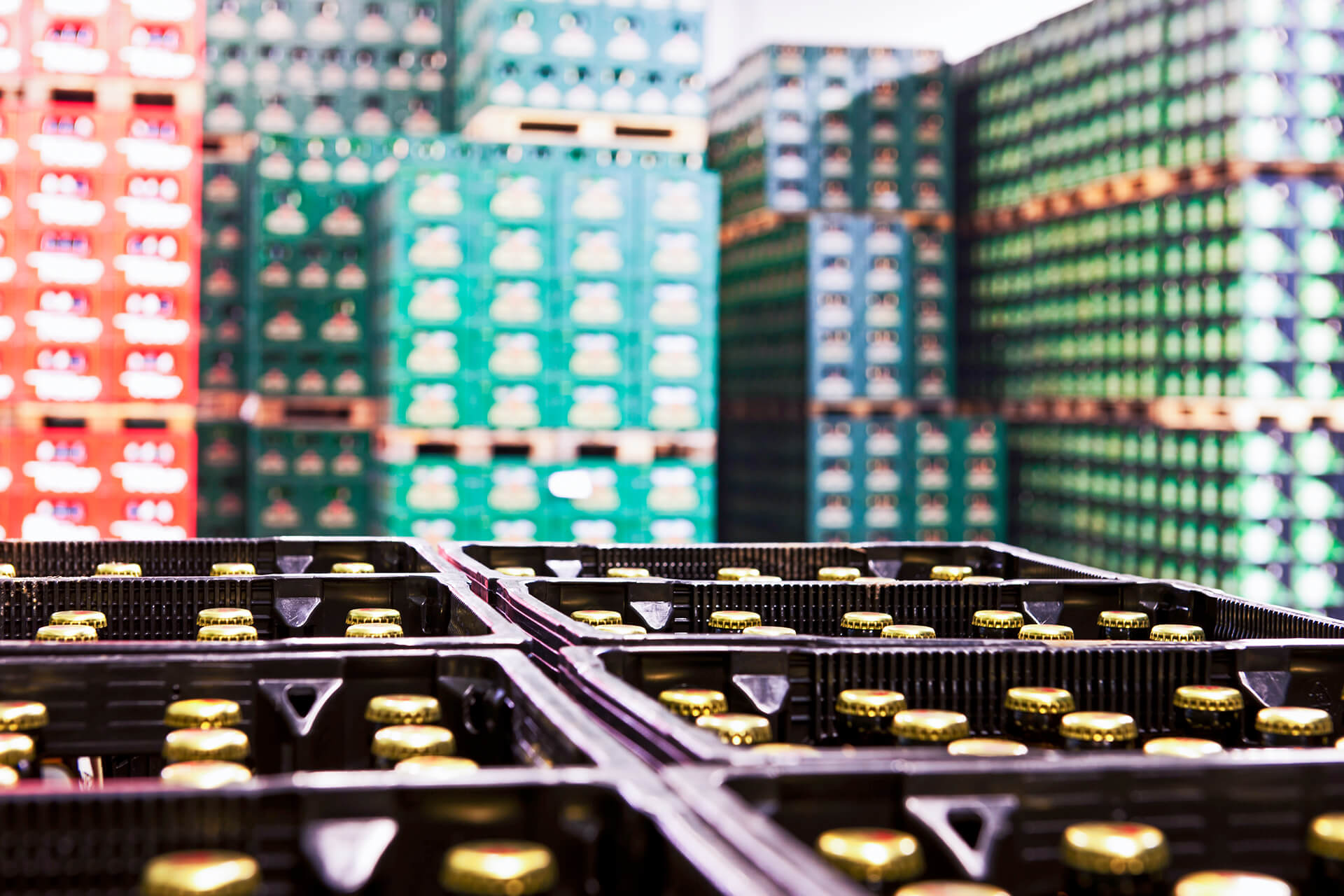
Solve complex sorting tasks easily
Machine Learning: How to intelligently address the growing cycle of empties
More and more new brands and products, increasingly individual bottle designs. The demands on optimally functioning and efficient logistics in the beverage industry have risen sharply in recent years and there is no end in sight. “For many years we have been dealing with the handling of empties logistics. We advise companies from the beverage industry when it comes to making their processes as simple and efficient as possible. This requires sorting systems with well thought-out and sophisticated technology that can cope with this complexity,” says Martin Brückmann, head of our Kassel site.
Intelligent recognition technology at the heart
The load carriers returning to the beverage logistics centres contain the most colourful mixes from all the beverage crates on the market or in circulation. The mixing of the bottles in the crates is no less colourful.
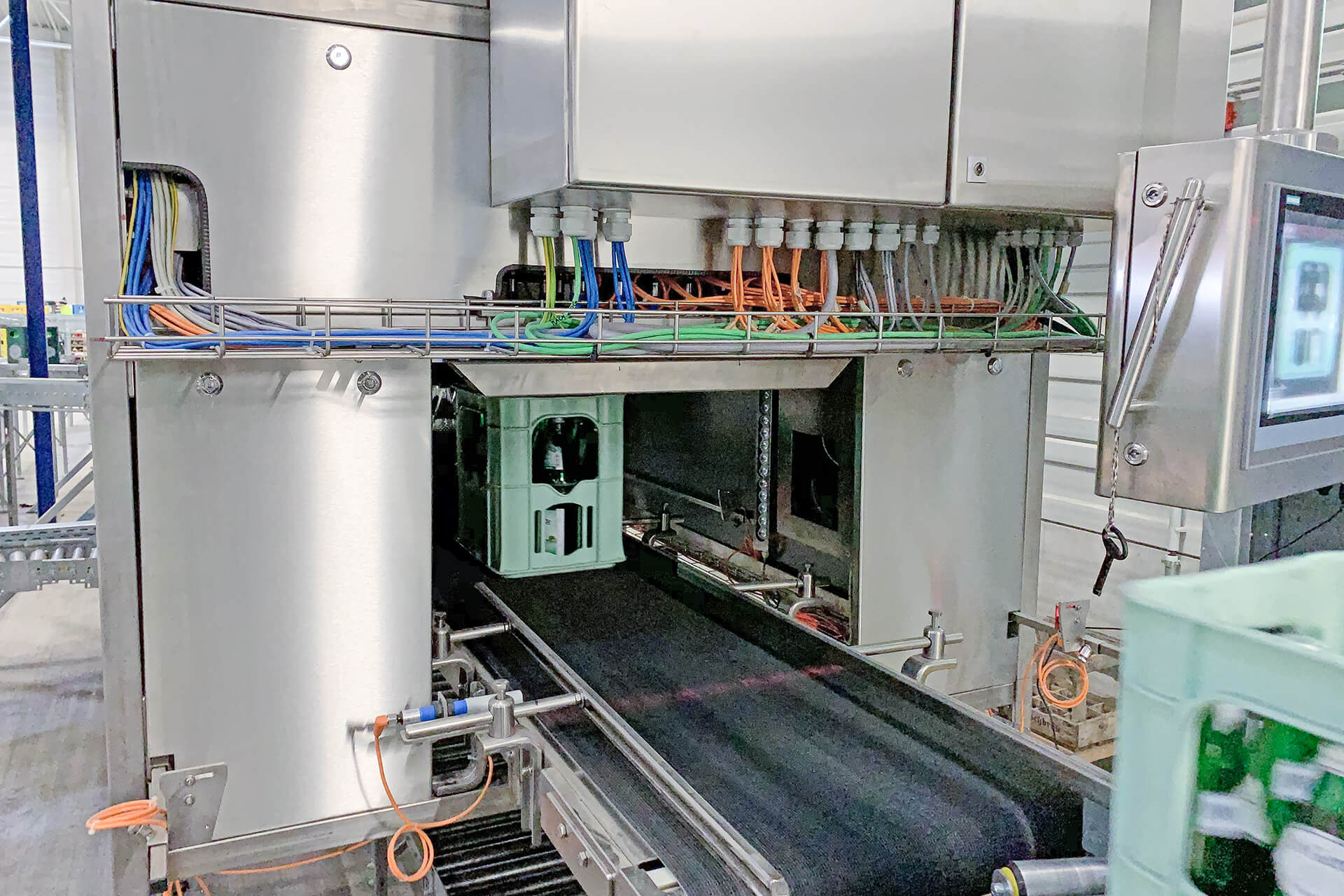
In order to be able to detect this variety of crate and bottle types in the shortest possible time and assign them to a suitable target, a reliably functioning identification is crucial.
We develop optical recognition systems for this task. They are the heart of every sorting system and function using state-of-the-art image processing. To do this, our SCHULZ Crate Inspector takes high-resolutionimages of every crate and every bottle, made up of many individual images. Our software works with these images and can fulfil every sorting wish imaginable.
Automatic teaching of new articles
Articles that are not recognised by the system are automatically ejected and must be subsequently maintained.
“It is true that new products can be added with the SCHULZ Crate Inspector,” explains our colleague Samuel Kees, Data Scientist in the R&D department at the SCHULZ site in Frankfurt am Main. “Nevertheless, this always involves a considerable programming effort, which drives up costs with ever new empties variants.” After all, each new item has to be recognised from a wide variety of angles, even if it is dirty or the label is damaged.
In his master’s thesis, Samuel Kees investigated how self-learning algorithms can be used to optimise this technique. The idea: to extend the Crate Inspector with Machine Learning (ML). Put simply, it is about mimicking and automating human learning behaviour in order to use it for specific purposes. Our colleague found suitable data with which the algorithm can train independently in his research work and was able to present extremely promising results.
The recognition rate is almost equivalent. An analysis of the few articles that were not correctly recognised also suggests that the incorrect recognition is due to a simple error in the data set. “Therefore, one can assume that the self-learning model will work even better,” he is sure.
Since then, it has been a matter of clearing the last obstacles out of the way and trying out the new technology directly with the customer. “At the end of the day, it’s all about how ML can be used in real time and how the continuously acquired data can refine our software.”
From theory to practice
Martin Brückmann was convinced by the extremely innovative approach right from the start. “For our customers in the beverage industry, the self-learning technology means a considerable reduction in workload for many processes. Not only is the time-consuming programming work no longer necessary.
We also expect faster processing and more efficiency. Another advantage is the permanent self-optimisation, i.e. the algorithm is always learning new things. But the commissioning at the customer’s premises is also faster. Fewer manual adjustments and calibrations need to be made.
In order to extensively test the practicality, our Frankfurt R&D team equipped a sorting plant with the new ML technology instead of conventional image processing. “The first tests are running as expected and confirm my master’s thesis,” says Samuel Kees happily and is sure that all the others will also work as planned. “But the decisive factor will be how the new process proves itself directly at the customer’s site. There are always situations here that you can’t foresee through practical pre-tests.” But he is confident about that, too.
Research and develop with direct reference
With SCHULZ’s own R&D site in the Main metropolis, we have ideal conditions for advancing new technologies. “Even large corporations often can’t keep up with that,” explains our R&D team leader, Sascha Desch, not without pride. “We don’t develop abstractly under laboratory conditions, but are directly integrated into customer projects from the very beginning, working very specifically with real data.”
Through this direct dovetailing with practice, we achieve maximum efficiency for our customers. This gives them a clear competitive advantage, innovation exclusivity and future security.
Much potential for further applications
Samuel Kees still sees a lot of potential for machine learning in other applications. Not only in the beverage industry or where recognition technology is needed. It makes ML sense wherever the complexity of the task makes classical programming work inefficient. And there are plenty of examples of this in the age of digital transformation.